Short-Term Power Trading: A Value Chain Perspective
energy-trading
19 Sept 2022
Alexander Reinhold, CEO
)
With rising shares of power generated by volatile renewable energy sources — sun and wind in particular — short-term power trading markets are growing strongly.
We categorize spot trades (i.e. Day Ahead and Intraday) and future trades made up to 2 weeks ahead of delivery as “short-term power trades”, implying that volumes and prices of electricity traded in short-term markets are primarily dependent on the weather. In contrast, prices for mid-to-long-term futures contracts are usually determined by macroeconomic and geopolitical factors.
The complex and highly dynamic nature of short-term power markets requires participants to process large amounts of heterogeneous data in real-time to ensure smart decision-making and efficient execution of trading strategies.
The journey from receiving the first data point to closing a trade is generally complex but can be divided into three major steps:
data processing
intelligent trading decisions
order execution
Let’s take a look at each step across the short-term power trading value chain.
1. Data
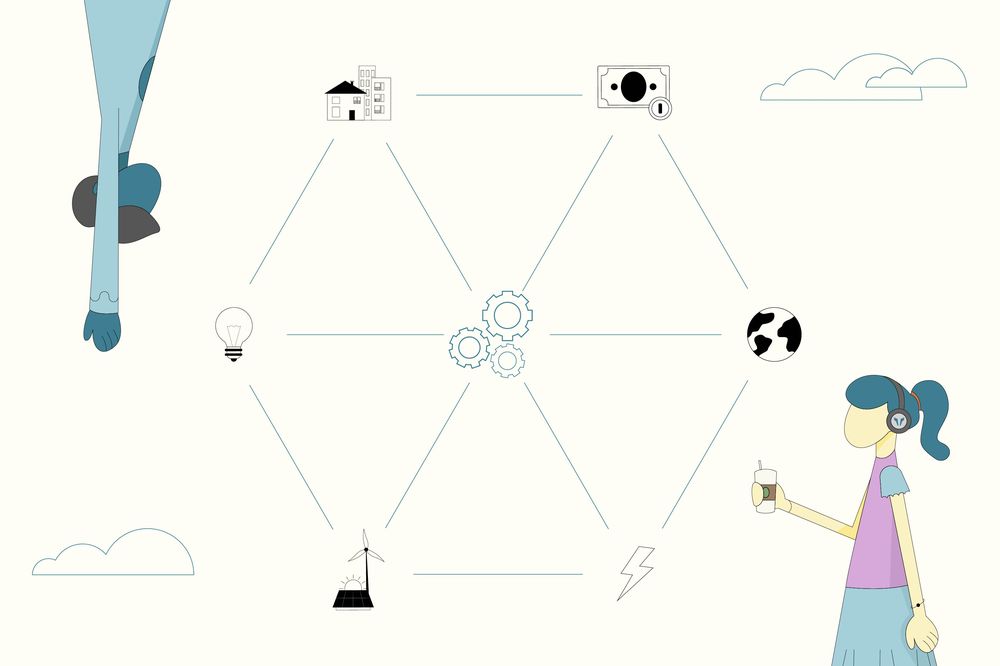
It all starts with data. In order to make informed trading decisions, one needs to process large amounts of technical and fundamental data from a variety of sources.
Technical data comprises historical trade prices, volumes and order books, which are turned into patterns to predict market trends. While technical data analyses are often successfully applied in extremely liquid markets (e.g. in stock trading), they usually fail to provide a comprehensive perspective on short-term power trading.
Instead, prices in short-term power trading are strongly driven by “fundamentals”— external factors, such as weather, that influence market demand and supply.
Fundamental data sources include:
Raw weather data — information about weather conditions in a specific region (e.g. temperature, cloud covers, precipitation, wind speeds, etc.)
Regional production forecasts and actuals (particularly for solar and wind production)
Demand forecasts and actuals
Balancing data such as grid frequency and balancing reserves activated by transmission system operators (TSOs)
Urgent market messages (UMMs) — e.g. large power plant malfunctions
Data points come in at different frequencies (usually somewhere between real-time and every 15 minutes) and depict different time resolutions. Processing large amounts of heterogeneous information simultaneously is therefore one of the biggest challenges in trading.
For that reason, a modern power trading company requires state-of-the-art time series data infrastructures, able to input and output data at extremely high speed and accuracy to facilitate intelligence and execution — the two subsequent steps of the energy trading value chain.
2. Intelligence
Intelligent trading decisions are the “holy grail” of power trading. Largely based on fundamental and technical data analyses, they consider three dimensions:
Whether to buy or sell
What volume to trade (e.g. buy/sell 10 MWh of power)
At what limit price to buy/sell (i.e. maximum defined buy price/minimum defined sell price of a given order — e.g. 100 € per MWh)
Intelligent trading decisions lie at the core of every successful trading strategy and have the strongest and most immediate effect on trading performance.
While the markets are becoming increasingly complex, an automated approach to deriving intelligent trading decisions is still rare in the energy industry.
3. Execution
Towards the end of a trade’s lifecycle, the performance of intelligent buy/sell, volume and price decisions is maximized by means of smart order execution strategies.
In so-called pay-as-cleared markets (e.g. Day-Ahead auction), all participants receive the same price, which is calculated using aggregated supply and demand curves. As a result, the only parameter that one can influence is the limit price. That is why the “Execution” step is only relevant in continuous markets (e.g. Intraday Continuous market), where trades are continuously executed every time a buy order price matches a sell order price.
Let’s suppose one made an intelligent trading decision to buy 10 MWh at a 100 €/MWh limit price in the Intraday Continuous market. A smart order execution strategy won’t place an order of 10 MWh at 100 €/MWh at once, as it’s uncommon to go “all in” in Intraday markets. Instead, profit is maximized by breaking down the 10 MWh into several sub-orders.
Similarly, gate closure is an important factor for order execution strategies: regulations require market participants to close any open position before the order book closes. For that reason, smart order execution strategies are aimed at closing a position rather than maximizing trading performance as one approaches the gate closure.
Other typical order execution parameters include stop-loss and take-profit limits, which are widely used in financial trading. Setting a stop-loss parameter minimizes one's losses to a specific limit: should the market go against a trader’s predictions, a trade will be closed automatically, not allowing a loss to exceed the defined limit. In turn, using take-profit guarantees an automatic trade closure in case the market price reaches a specific profit level.
For example, one is selling 1 MWh of power, setting a take-profit at 120 €/MWh and a stop-loss at 90€/MWh. If the prices suddenly go down and reach 90 €/MWh or lower — the trade will be closed automatically at 90 €/MWh. Alternatively, if the prices reach the take-profit limit — 120 €/MWh — the trade will be closed automatically, bringing 20 € of profit.
Verdict
Unlike other trading markets (e.g. financial, crypto or ad exchanges), power trading is still primarily subject to human decision making.
However, recent changes in market dynamics are making manual trading less and less feasible: an increase in decision-making speed and growing data complexities will likely lead traders to seek more technology-based approaches in the future.
As a result, many traders have upgraded their data processing suites and started making use of computer-assisted trading to optimize order execution in recent years.
However, the complexity associated with tomorrow's energy mix (i.e. high volatility due to weather dependency and more flexible assets like electric vehicles) and the market’s growing reliance on real-time data processing will leave less and less room for data infrastructure delays, human decision-making and imperfectly integrated third-party trading applications.
Instead, modern time series data infrastructures with real-time data processing engines, fully automated trading decisions and integrated order execution software will pave the way for the future in short-term power trading.